For the 1st episode of FuseBytes Season 2, our host Nate Rackiewicz is joined by Vijay Venkatesan, Chief Analytics Officer at Horizon Blue Cross Blue Shield of New Jersey to discuss the fundamentals of AI readiness. Tune in to learn about ensuring data maturity and operational readiness, building a lasting AI culture, lessons from industry leaders and much more.
Introduction
Nate Rackiewicz: Well, greetings, my friends, I'm Nate Rackiewicz, your host for FuseBytes. It's a podcast brought to you by Fusemachines where I'm serving as the EVP and Head of Data and Analytics for North America. I'm really excited to be with you here this week talking about AI readiness and companies, which is the topic of this year's focus of FuseBytes.
It's a new season of FuseBytes. We're gonna have about 10 episodes in this season. Really excited to get started today with episode number one that will focus on understanding AI readiness, the foundations for success. Why, AI readiness?
Well, we hear all of the hype out there about artificial intelligence. You can't get away from it. I was just this weekend up in Pennsylvania talking with my father in law, and all he wanted to do is talk about artificial intelligence, too. It's at every conference you go to. It's been this explosion of interest since ChatGPT burst on the scene about a year and a half ago, and we just can't get away from it.
"So you'd think with the prevalence of artificial intelligence out there that it would be easy to implement within companies. Well, the truth is, it's very challenging. In fact, one stat that I've seen says that 85% of all AI projects in companies that are just getting started on their journey fail."
And so what we wanna do with this podcast is really bring together thought leaders, best practices, tips and tricks that will allow you, our guests here, our audience, to get to success faster on your own AI journeys within your companies. That's the goal of the podcast, that's what our focus will be this season on AI readiness and companies.
And I'm really excited to start episode one today with our guest, Vijay who is the chief analytics officer at Horizon, Blue Cross, Blue Shield of New Jersey. We go back a number of years. So I'm really excited to see him here again. How are you doing, Vijay?
Vijay Venkatesan: I'm doing great, Nate. Thank you for having me on. Looking forward to our conversation today.
Nate Rackiewicz: Excellent. Me too. So this explosion of interest in artificial intelligence. You've been riding the curve of this for many years. And you know, from data to analytics to artificial intelligence. You've served as a chief data officer at a prior company. Several Vice President roles in the healthcare industry. I'd love to hear about your background and your journey to becoming the chief analytics officer at Horizon, Blue Cross, Blue Shield of New Jersey.
Vijay Venkatesan: Well again appreciate the opportunity, Nate. And you know I've had kind of an interesting career into the world of data and analytics. I began in a much more of a traditional IT background, you know, thinking about large scale application systems. And how do you implement them to be able to meet the needs of an organization. But over the years I think what intrigued me was not about the application itself, but what is the content in the application. And how does that bring meaningful value in the context of both business operations, or from the perspective of customers, if you will.
“And that got me passionate about data and specifically data and healthcare, because, as I've gone through my years of experience, the thing that always intrigued me is how do we use data to make real, meaningful impact in human lives? And that's been kind of my passion over the last 20 years.”
which is really about using data to get insights, to be able to then drive meaningful action that impacts human lives at the end of it all.
And that's been my 20 year career of sorts in healthcare, and how I ended up at Horizon was, you know, they were looking for an individual to come in and be able to transform the use of what I call health insurance data to be able to work with our providers in the State and be able to impact, you know what we call our value based care or really population health at scale.
So it was the art and the science of bridging the data, the analytics, the insights. But then to be able to say, How do I impact or influence the care that a patient receives at the end of it all, and that's been kind of my lifelong passion. Because I wanted to be a doctor early on. I said if I couldn't be a doctor. The next best thing is to be around the doctors and impact, you know, patient care. And that's kind of been my 20 year roadmap of source.
Nate Rackiewicz: It sounds like a great purpose filled journey as well, which is really great to hear. You know, when you can find that meaning. They say you never work a day in your life right?
Vijay Venkatesan: That's absolutely the fact. I mean, I always say that you know when people ask me, how would you define yourself, you know, and I always say to myself, it's about creating positive change.You know, one individual at a time, and I think that's really kind of been the mission for me in healthcare.
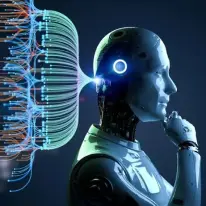
Transform your business with AI and Data today!
Book a callNate Rackiewicz: So did the chief analytics officer position exist before you stepped into it? Or was it a net new position at Horizon, Blue Cross, Blue Shield of New Jersey.
Vijay Venkatesan: The position did exist when I joined Horizon Blue Cross, Blue Shield, I think what they were looking for is maybe a slight, different orientation to it. So when I came into Horizon, the objective of analytics was, How do we serve our individual customer segment? So that's operations across the health plan or a health insurance company. And what they wanted to do was to say, how do we make that more efficient and optimized? And at the same time. How do we then take that to our provider partners and really start impacting patient lives?
So this idea of having somebody with a provider background to come into a payer industry and be able to say, what are those intersections, and how do we use data more intelligently, more effectively, and in a more proactive way rather than a reactive way. That's how I ended up at Horizon. And so part of my role there has been to say, How do you advance the cause of data, but also use intelligence in a meaningful way that does drive meaningful action at the point of care. And that's kind of been my 5 years or so at Horizon to be able to advance that at scale.
AI readiness essentials: Data, infrastructure & support framework
Nate Rackiewicz: That's great. And it's really fascinating to see your journey. You know, coming at this analytic side from the data angle originally in your career. Which is the same journey that I took in my career from the data side onto the analytics side onto the artificial intelligence side and to ride that curve has been really exciting from a career perspective. I know it has been fulfilling for me as well.
So a question for you as we think about AI adoption. And we think about the failure rate that's out there for companies that are trying to get started with this? How do you think about a foundation for success in AI adoption when there are so many challenges that companies face in trying to implement it?
“No, that's an excellent point, I think. Part of when people think AI, everybody has what I call their own definition of AI, and so the hardest thing to do in this conversation is to come at it from a point of view of how do we do this responsibly and ethically. Part of the conversation also has to be where do we apply? When should I apply? And how do I apply?”
So for us, the foundation was really focused on establishing a governing model, and the idea of the governance was, How do we define you know our rules of the road, if you will, around ethics, around where should we apply it? And because we're a healthcare company, it's really about protecting the patients data and making sure that we're not doing something we're not supposed to. And how do I answer those simple questions of can I? And should I?
So that means. We started with the idea of an AI Governance Council, and the goal of this Council was to say, What are the right use cases? How do we evaluate the risk portion on these use cases and say, Is this a high risk? High risk, meaning? There is an opportunity for looking at the patient data? Have we taken all the protection and guardrails? Is this the right use case? And should we even use it in this way?
So answering all those questions first, before deciding, should we get on the AI bandwagon, if you will. So that was very critical for us, being a healthcare company that start with responsibility, start with governance and create that governing council comprised of our privacy, security, compliance, and some key business stakeholders along with our IT Colleagues to say is that an effective way to look at the use case from all sides, and be able to then arrive at a risk score which then informs of the next steps.
Fostering an AI-driven culture: Encouraging experimentation & learning
Nate Rackiewicz: You just talked about a number of the stakeholders that are involved, that are, I imagine, at very different levels in the organization. I wonder what role does culture play in fostering AI readiness within your company?
Vijay Venkatesan: No, it's a wonderful thing to ask, and it's a difficult question to answer. And part of the reason is because there are 3 sub cultures in every organization. And so there is an executive view of culture, which is, they've heard the hype. They've heard the buzz. They want to do something.
Then you've got the folks who are doing the everyday operations and they are more concerned about, how does this impact me on a day to day level? Is it gonna take my job functions away? Or is it really gonna enhance my job functions?
And then you've got folks right in the middle, who are trying to balance between, Where do I apply this in a meaningful way that I see the business value being realized and so balancing the culture at all these levels is going to be important. And that starts with what I call education for each of the different levels.
So we call it, like the 3 layers of how we look at AI, the first layer is really focused around, Can I use it to just make my processes more efficient? So that's more at the rank and file level to say, can I make your job functions easier to do so? That's like productivity improvements that I can bring to you, any assistance I can bring to your augmented intelligence, that'll make your life easier.
“At the executive level it's almost all about how do we advance? How do we pilot and test and advance and show what I call business value that really translates into changing the way we do work. So that's like reimagining our work, if you will.”
And then the folks in the middle and we're all trying to figure out, Do I work at the edges of AI? Or do I make AI, the epicenter of how I do my work? That may be it taking a longer turn, if you will, in terms of time Horizons. But if you can get the executive view and the rank and file view aligned, the work in the middle is really about, how do you shift the focus from, you know, doing the way we do it today to reimagining a future that's possible.
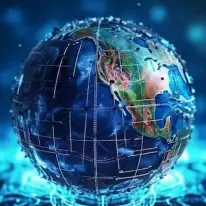
Leverage our AI products and solutions.
Schedule a meetingNate Rackiewicz: That's great. Really interesting to hear how you approach those 3 different levels that you described from a culture perspective, I imagine that the section in the middle might be the most difficult, because they're they're torn between the culture of the executives and the culture of the rank and file so I imagine there's there's a lot of activity that happens there.
Vijay Venkatesan: Yeah, my simple analogy used to be being in the middle is like being the middle child and a family. The oldest gets all the attention for all the wrong reasons. The youngest gets no attention, but can do whatever they want. The one in the middle always feels like I didn't get the best of the both worlds. In some ways people in the middle have to figure out. What do I do with this toolkit?
And I think that that's the other important aspect of this conversation is not to think of AI just as another new thing. It's like it's another tool in the toolbox. And how do I think about it in a way that both accelerates my transformation or advances and innovation? But at the same time allows me the time to go through the people process technology in a meaningful way. I think that's the balance. And that's why it takes time.
Nate Rackiewicz: So what do you think? I mean? This sounds like a very reasonable approach to setting up your foundation for success, for AI readiness. What do you think are the primary reasons behind the high failure rate of AI projects within companies?
Vijay Venkatesan: I think the high failure rates is because in general I mean I don't know if you recall, you know, when we were, I would say when I was a lot younger. I remember when the data warehouse was the biggest hype, you know, like everybody wanted a data warehouse or a data mart. And when you look at those early years of data warehouse builds, there was a high degree of failure in those.
And the answer was, it's because we didn't quite understand what the requirements were for. You know what type of analysis people wanted to do, and are the questions that they're asking, are the answers going to be consistent because we don't have the right definition, the right framework, or the right data itself?
I think the failure rates in this conversation is very akin to that which is, do I have the right data?. because a lot of people forget garbage in garbage out used to apply in the data warehouse paradigm. It applies in the AI paradigm as well.
“Your data isn't AI ready? Then the algorithms can only do so much, and the insights or the output is going to be not as helpful.”
So when you think about those paradigms, I always go back to 20, 25 years ago and say good data still matters. So you need to have AI ready data. Good governance still matters, which is, what do I do? And how should I use it? And what are the guardrails? And then the good infrastructure, which is, where does this run on? And how does it run on? And how do I know that it's industrialized and scaled in a way that's gonna continue to evolve and build as I go?
So those are all the same paradigms that people tend to forget because they think all I need is to buy a large language model or partner with somebody to get a large language model, and then the magic happens. The magic doesn't happen. The hard work is still the hard work, the notion of governance and data is still the same. I think that's what people forget.
And in my experience, that's what I've seen, which is in order to get AI done right, you need to have the right data, the right infrastructure, the right security, the right support framework and the right, what I call the distribution model. So if you think of it in very elementary terms. It's the, you know, AI ready data. AI ready Ops, with an AI to gateway that helps you to do things in a secure fashion and distribute the outcomes.
Strategic decision-making: Buy vs. build in AI implementation
Nate Rackiewicz: So it sounds like another set of foundational elements right there. That can lead a company to success on these things. How do you think companies should prioritize their AI initiatives to maximize success?
Vijay Venkatesan: It's a great question, and I talk a lot about what I call organizational maturity, and I think one of the things that I always say is, that is your organization prepared to take this on in a meaningful way? So the way organizations come at it is, including ourselves, we do a lot of pilots right in general.
“With any new technology or paradigm, there are always a lot of pilots. As you do these pilots you have to be crystal clear about what problem you are trying to address with each pilot. And if you don't, if you're not clear, then I think what happens is you have a lot of R&D, but not enough things that can blend itself to moving to production."
And I think one of the most significant stats in AI has been that, you know in the last year. If you look at how many projects have really gone to production. That number is in the low single digits.
If you look at across the industry segments, and the reason is is because you people are doing too many or too much of R&D, and not enough of what I call a structured approach to making this a true SDLC, or how do you do a software development lifecycle model for GenAI use cases? And so my question, first, to most organizations would be, are you AI ready? What is your AI maturity?
And the AI maturity, you will see, follows your data maturity. So if you've done any data maturity roadmaps that will inform you of your AI maturity as well.
“There's no such thing as AI maturity outside of data maturity — those go hand in hand.”
So I would encourage companies to think about their data maturity roadmap, and say, Where are they which will then get, give them some insights to. Are the AI ready? Which then you can it? You used to say, what pilots can I do to either advance or move the needle to get to where I need to be on a maturity paradigm.
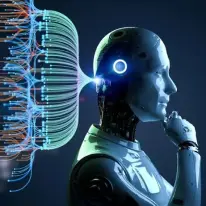
Transform your business with AI and Data today!
Book a callNate Rackiewicz: It's interesting that you brought up the SDLC. The SDLC, I started my career as a software engineer and rose up the ranks at HBO. As a software engineer, I became a VP of the application development group there and saw a strong, really strong SDLC process that was in place from a software engineering perspective.
When I switched over to the data and eventually data science side, I saw a lack of SDLC and a lack of discipline around software management best practices and a big opportunity to bring that to the data science realm and then from there onto the AI realm. So it was interesting to see how companies are, and this different skill sets, we're taking a step back in the areas that we're going to advance the companies. And we still see a lot of lack of discipline around SDLC in many cases in terms of how AI projects are structured.
Vijay Venkatesan: No, it's an excellent point, and I think it's something that when I came to Horizon one of the things that I noticed was data science was in vogue. And so everybody was doing it. And we had like 40 different projects we're doing on data science. And I simply asked one question: Who is the operational owner of this use case and are they prepared to apply the insights from this data science algorithm tomorrow morning when we're done with it?
And the question was, we don't know. And
“I said, I think we're gonna stop. We're not gonna do R&D, we're gonna take 9 months or 6 months to hunt for the right use cases where there’s operational readiness to impact in a meaningful way. That's how we defined our data strategy, which was data to insights to meaningful action.”
And I think if you think of that and apply it to your AI framework. It's the same idea: data to insights to meaningful action. And you have to make sure that there is an operational readiness component. And what that means, then, is to your SDLC comment is, you have to have a process, a process by which you'd gather requirements, process by which you do your model operations, process by which you then deploy the model. How do you manage the efficacy of the model? Then how do you know that it's hallucinating. How do you know that it needs to be either modified or you need to build a new model.
All those disciplines you applied in data, science applies in this world of LLMs, too.
“What people forget is it's still a process, and it still requires a structure. And my worry is that Gen AI will become what data science used to be.”
And then came operational processes like ML Ops. So you need an AI Ops or LLM Ops, If you will still defined.
And if you don't think that way, you're gonna find yourself in a paradigm where you'll have a bunch of things running, and you're not quite clear on, how do I manage and maintain? And companies want to understand? What's the support model requirement for this longer term once it's, you know, industrialized and operationalized. So we have to bring what I call the Ital discipline to this conversation.
And those are the things I remind people of is that, that's why I use the term tool in a toolbox. Because if you don't think that way, you will think that this doesn't require an SDLC or it's not important or it's not critical. You know, the machines will do it all for me.
Machines will automate, machines can do a lot of monitoring. But you still need a data ops process, a data observability process, a model of observability process. You still need to figure out what's the human element in this equation of hallucinations? And how does that work.
All of those still matter. And so I stress quite a bit. And maybe I'm old fashioned, as I always say, that the process matters, the structure matters, the model matters. It's not just about intelligence for intelligence sake.
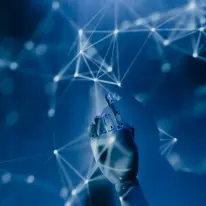
Leverage AI to elevate your operations and gain a competitive advantage for your business.
Book a meetingSharing use cases for AI implementation
Nate Rackiewicz: Yeah, it's really interesting. And you know, I'd love to if you could share some case studies or use cases where you've seen AI prove successful or those that have met the challenge that most initial AI projects face.
Vijay Venkatesan: Yeah, no, it's a great question. And I think one of the things that we've found. And I think the way we have defined what I call our starting point for AI use cases is find things in your organization that you think are mundane and repeatable because there is not a high risk there in terms of. So if you're looking to your help desk as an example in your IT shop, and you're trying to say, hey? I get asked the same 10 questions of How do I do something?
Those are all in your standard operating procedure somewhere, and you know, instead of opening a help desk ticket, ask a question. How do I make it more interactive using? Maybe you know teams, you know, Microsoft teams as a front end cause, that's our collaboration tool and answer these questions quite easily to anybody in the company. Right? How do I open up that kind of an intuitive interface to start to engage around standard operating procedures.
So that's one of the use cases that we are attempting to do as we speak is this notion of what I call intelligent chatbots. But you really using your collaboration tool as the front end to it. So that's a simple use case where you could say it can call the millions of, you know, pages of documents. I say millions. It's not that bad. It's probably hundreds of your standard operating procedures and really kind of call the information that people are looking for where you can start to showcase to people. Hey, this is how we can improve productivity or efficiency in our, you know, or within our 4 walls.
The other things are we're looking at ways. How do we enhance our member experience, you know, for a lot of questions members have on their benefits, if you will. How do I answer that? In the language of choice of the members?
Today, we print a lot of manual handbooks in each language is there an opportunity to both produce paper, but at the same time make it much more of an enhanced customer experience, if you will or member experience. So we're looking at PDFs and say, can I answer these basic benefit questions and point them to the page in the benefit handbook, where they can get more information, so simple things that will start to show people that intelligent automation has a place self learning model has a place and start to show how it can improve productivity, efficiency, and engagement at a much more scale that it's harder to do today with just people.
Nate Rackiewicz: So if you're focusing on the mundane tasks and the repeatable tasks and thinking about other use cases that you could apply artificial intelligence to, how should you think about, or how do you frame ROI in starting the AI journey?
Vijay Venkatesan: I think you have to almost think of the ROI conversation independent of the piloting efforts. And the reason, I say that is because you have to do a few trial and errors to say what is the right large language model? What's the right infrastructure? Who's my right partner? There's a lot of what I call kind of building the house, or it's almost like, what's the Blueprint for the house. So if you think of large language, model or architecture patterns in 4 buckets that there are things that you can buy. There are things you can customize, and there are things you can just build from scratch.
And there are different buckets that you have to go through to figure out what's my right model for my organization, and you will see that every organization will say I may need all of it. I may want to buy a Blueprint because, It gets me off the ground and running.Then I may need to add an addition. And so that may be more custom addition I want to do, because it's very unique to my organization or my industry. That's as a lot of you know, security privacy components, I need to add. So I think what you're gonna see is a Horizon.
And the Horizon, says, How do I start quickly? Which may be just a buy model which really means is that it's working with your existing vendors or technology platforms you already have, and say, can I adopt what they've already in built for me? So if you're a customer who uses work day as an example or service now. They're already building some of these intelligence in their products. So you may say, I want to go to the next version of that software, and that gives me, you know, step one towards this AI journey. Then you may say, Hey, I may want to. You know, partner with Microsoft, because I like what they've done with co-pilots, and I want to use that to be my customer. Help desk and start to use that as a help desk framework and maybe take their model and customize it.
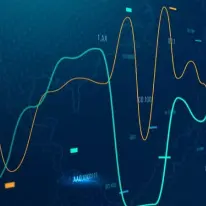
Discover how your business can grow and transform with AI.
Book a meetingVijay Venkatesan: And then another case, you may say, no, nothing fits that's available, and I really need to invest. And so you'll find organizations kind of vacillate between these extremes and just trying to figure out what makes sense from a cost, quality and an ROI. So
“The ROI discussions can be had once you identify which direction and how far down that direction you're going to go, and what use cases? And what's your intent? I always say that you need to be clear about intent.”
If I'm just trying to reorganize my process within I key as an example. The Roi will be smaller. It may be a 25% productivity improvement if I'm trying to reimagine my process that may be 50% as an example. But if I'm trying to cannibalize my own product and company and create something different that may be significant, but it also requires a significant culture shift and a commitment shift to get there.
So you almost have to say, am I killing my company? Am I killing my department, or am I killing my process to get to an outcome, and when I say killing you can replace it with reimagining. Am I reimagining my company? Am I reimagining my department? Or am I, reimagining my process? And that's how you have to kind of think about these things. Otherwise I think you'll get. There's no simple answer, like, there's one LLM, that does it all. There are many LLMs for many different uses and then you have to make those decisions appropriately.
Nate Rackiewicz: What I found is that some of the use cases, close to financial forecasting, tend to be ones that you have a higher chance of demonstrating ROI and being able to get a number tied to it. That's just what I found in my own history. Across different companies. And so that's a place that I, you know, get in and advise companies to think about starting. Because if you can forecast better. You can get to predictive analytics faster. In that experience from an ROI perspective. I.
But that's not where all AI happens. AI happens across these companies and I've experienced and seen and you know the trouble that some teams go through and getting that buy in without that direct tie to the ROI so I imagine it goes back to the culture that you were talking about before, of the willingness, and the having champions that are accepting of the fact that there may not be a clear path to a direct link to ROI, but to give you still the, the, the flexibility to run those pilots.
Vijay Venkatesan: Yes, and and I think this is one discussion where I think maybe starting with ROI, maybe a nonstarter, to begin with, because I think what you have to show is show possibilities and the best analogy I use is that you know, when the iPod came out, you know, years ago, it feels like now. Nobody needed the iPod, you know. You could have made the argument that, hey? We were happy listening to it on our Walkman then CD players and then that was fine, like, you know, we're happy with that type of an approach.
And then came the iPod, which said, We're now digitizing all content. And the way to consume the music part of digitized content was the ipod. And then it evolved into the iphone, where now it's not just a phone to make calls. It is kind of an extension of you with your whole life stored on it, including your music, by the way. And so that became the next.
So in some ways the GenAI discussion will follow a similar pattern. I think this is again just my humble opinion. I could be wrong. I could be right. Time will tell, but I think we're in that same boat, which is we're trying to figure out– Am I building the ipod with the version 1.0 of this conversation, or am I getting to the iphone itself. You know, and I think that is a different cultural shift.
“Going from flip phones to iPhones was a shift, going from CD players to iPod was a shift. But that's the shift organizations need to go through. And that's where culture plays a big role, If you are the last person who still has a blockbuster membership, you're gonna take a while to get there.”
Nate Rackiewicz: Still have a lifetime membership to Erol’s. If you remember that.
Vijay Venkatesan: Exactly, so those are the ways I look at culture, right? Each organization makes that choice and it requires different mindsets to change and also opportunities. Right? Like, I think that that's the other thing we miss is like, if there is a new opportunity. And this tool can be that maximizer of that opportunity. Then you will see people start to embrace it. So I'm more optimistic about this, which is that I think this is where you'll see an opportunity. Intersect the value, and that's how ROIs will get built over time. Otherwise it's going to be a slow grind of sorts.
Cultivating partnerships: Leveraging external collaborations for AI success
Nate Rackiewicz: Yeah, I think the key is also just ensuring that the used cases are aligned with the business strategy. And that there are clear business questions that the business is asking, that the AI initiative is set to answer and gaining that buy in from mid level management up to senior management is key, and the more that you can identify and align with the business questions that they're asking the more likely that it is that they'll also, you know, give you the buy in and champion the work that you're trying to do.
Vijay Venkatesan: Yeah, I think one of the things we've attempted to do is to not use the term artificial intelligence. But say, augmented intelligence. So in some ways you almost have to say, is this a companion tool? This is a companion to us, and that's the way we want to frame it right like I always you know, when I use like Alexa, for example. Even though it's omnipresent in your homes. But it's still viewed as a companion suite or a companion tool.
And I think that's the notion we need to bring into enterprises is think of it as augmented intelligence. It doesn't remove the human in the loop, but it makes the human more valuable in the loop and I think that's the distinction you have to make. And most organizations don't start that way. They'll say, AI is gonna replace my workforce X or Y or AI is, gonna make this faster and may not need the human in the loop.
The way we have approached it is that it makes the human far more valuable in the loop and gets them to focus on the right things in the loop, and this is just the means to the end, and maybe a superior means to the end. But it's still a means to the end. And it doesn't devalue the humans in the loop. And I think that story is very essential.
“If you're trying to change hearts and minds within organizations, you have to know that it's about the humans in the loop.”
And without that discussion it almost feels like everybody, is a commodity, and and and you and I well know. Even if you're viewed as a commodity, each of us brings something unique to this conversation in the way work gets done, and I think that shouldn't get lost in this conversation.
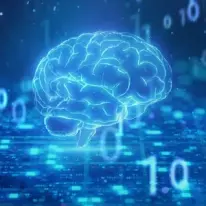
Leverage AI to elevate your operations and gain a competitive advantage for your business.
Schedule a meetingNate Rackiewicz: So is the journey that you're on and the journey that companies are on when they want AI adoption. Is this about building an internal capability? Or do we go back to the classic, buy versus build mentality where there are external service providers that could come in and help accelerate the journey for companies?
Vijay Venkatesan: Yeah, no, it's a great point. And I think it depends like, I've seen you know, some of the hyper scalers investing because they can, and they should, and they must. And that's one side of the spectrum, I think, traditionally like in organizations. I think what's more common, or, what may be more apparent is, I think your goal is to buy the capability because it helps you accelerate cause for you to stand up server form with NVIDIA Chips and GPUs is almost like unthinkable.
I think there are people who do that well who have done it well, and I also think you're not going to apply this technology universally everywhere you're gonna pick and choose your spots. And so there may be vendors specifically who honed in and and have done the R&D to be able to say, How do I do this particular function really well, and so I think you're gonna see that it's gonna be more by initially or by. And customize, maybe is the best way to say it because of some unique needs. Your organization has very few will do the build, and the people who are doing the build are gonna be companies like Microsoft, Amazon and Google, and many, many others like Work Day and others, but even they are buying and augmenting versus building from scratch.
So I think you're gonna see that between what I call producers of AI and consumers of AI and majority of the consumers of AI will have some version of a partnership. That they're gonna advance. And I think you're also going to see a lot of what I call collaborative coming especially in healthcare, where it's not gonna be like one company doing one thing they may want to pool their resources to say maybe 3 of us come together because we're in contiguous geographies, and there's more economies of scale if we can all come together to solve a particular problem.
So you're gonna see some of those collaborations start to form as well. So I think you're gonna see different different what I call initial models of all before it finally kind of resolves itself. As to are you on a buy site or the build side. My humble opinion is you're gonna buy initially and then, as your maturity happens with the platform and technology, then you may see a degree of customization occur.
Nate Rackiewicz: Yeah, that the approach that I took in my prior roles I served in enterprise roles as chief data officer like yourself and other heads of data, science and analytics. And that's what I did. I brought in external help to help accelerate the data and AI journey. But with the goal of internalizing certain capabilities that were core to the business over time just because it took it took time for internal staff to develop the skills and the competencies necessary for successful AI adoption.
Vijay Venkatesan: No, absolutely. And I think one of the other things I forgot to mention was that when you think about AI Ops, you have to understand is that your IP that you're you know putting out there? Or is it really the commodity that you're putting out there? So we truly believe that if it's your IP, you should do everything to keep that kind of internalize it as much as you can with the right, you know. Model if you will because those things are all what I call is intrinsic to who you are as an organization, and you don't want to be just outsourcing that in a meeting, you know. I would say, like outsourcing your IP may not be the right longer-term answer.
Nate Rackiewicz: So we have an audience here of C-level executives other executives as well. Decision makers. You know, if you're in their shoes. What are the critical components of a comprehensive AI readiness, strategy? What are those key components that you would divide this into.
Vijay Venkatesan: I think, in terms of readiness, I would say. Understand your data strategy and your AI data readiness. First understand your partner landscape and see who brings what? To whom? For you know in context of your operations. How does that align to your business strategy and then pick. What I call low risk areas and pilot because at the end of the day what you're going to find is data is still at the epicenter of this conversation. You have to know what data you have structured unstructured, semi structured, audio, video.
Whatever the data contents are making sure there's a way to catalog, have that AI prepared if you will. The second piece is really the understanding. Your partner, landscape, and what you have and making sure that are there areas that where you can align better because it aligns to a business strategy.
The overarching theme I began the discussion with is still about having a governance model and starting and creating that first. So I'm almost assuming that that's going to be there, because without that everything else becomes harder to do, having a governance model to start off with, but having AI ready data.
“Having the right partnership for framework, doing some pilots and picking low hanging fruits in terms of business problems that can start to showcase both the value and the gaps that you have within your organization, will help inform and mature your AI strategy. Then you can say, here are the big things we can start to tackle.”
Nate Rackiewicz: That's great insight. So, that's really a lot of great insights. Tips for success. You know, there's all those obstacles that you hit you talked us through. How you deal with some of those. I'm wondering if you could provide some examples of companies that you like that have successfully transitioned into an AI ready organization.
Vijay Venkatesan: I think, without naming particular companies. What I would say is that in general the banking and financial industry segment has done a pretty good job I think they were always been early adopters of AI, even when it was just called data science. And now, when you think about generative AI. They're looking ways to help optimize, be more efficient, be a better admin at customer engagement, be able to create new product offerings by understanding customer segments better, portfolio is better, I think banking and financial is who I look to.
So that's one area I look to the other is the the consumer product goods in general, like they've done a great job as well in terms of supply chain management, inventory management, being able to know what products to stock, not stock. I mean all those type of things, you know, just the basic running of stores. And how to get intelligent fleet movement, how to use and track inventories across from the time they're on a wholesale to what I call that the the the store model. So there's a lot of industries that have done well.
I think what we have to be careful of is saying just because it work there, it automatically work will work in my company. And so my advice to everybody has been, learn as much as you can see what framework works. But understand, where were those organizations in terms of their maturity. Both in culture as well as data? If you understand those 2 pieces, then then you could say, What is my roadmap going to look like? Is it a 6 month journey? Is it a year journey, or is it a multi year journey.
And it's very important to assess that because sometimes we make the mistake, that oh, it works with my the bank, so it must work with my customer. And out there those are 2 different customers with 2 different goals and objectives. So we are very mindful of that. And sometimes I feel like people tend to fall into that mode of saying, Oh, my! You know my! My grocery store is doing this, or my bank is doing that. Therefore my customer will embrace it.
You know, and it's not the case. And so you have to be very careful about what is it you're in the business of what matters in that business? How do you make sure at the end of it your brand loyalty or customer loyalty doesn't get impacted because that's the other aspect that people don't think about is the downside risk of using AI. Like, what are the risk? Factors that may there be there, both from your but brand your loyalty, all of those aspects which are equally important.
“That's the other aspect that people don't think about is the downside risk of using AI. Like, what are the risk? Factors that may there be there, both from your but brand your loyalty, all of those aspects which are equally important.”
Nate Rackiewicz: That's great. And with that we're gonna wrap it up, Vijay. I'm so grateful that you joined me here on the first episode of FuseBytes for this season where we're focused on AI readiness in companies. Today's topic was again understanding AI readiness, the foundations for success. So thank you, Vijay.
Vijay Venkatesan: Thank you, Nate, and appreciate the opportunity. I hope you have a wonderful 10 episodes in terms of learning from others. I was honored to be the first guest, so thank you for having me on.
Nate Rackiewicz: Sounds great, and to our audience, thank you for tuning in and stay tuned for new episodes of FuseBytes where we're focused again on AI readiness in companies. I'm your host. Nate Rackiewicz. And this program is brought to you by Fusemachines. Thank you!
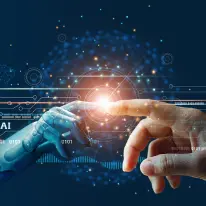
Partner with Fusemachines & unlock the transformative power of AI.
CONTACT US TODAYKnow our speakers
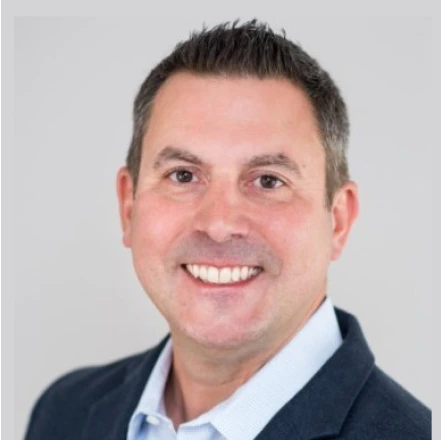