In this episode of FuseBytes, host Nate Rackiewicz talks with Scott Taylor, The Data Whisperer, about the critical aspects of data trustworthiness and integration. They explore the challenges of evaluating third-party data, the importance of data coverage, structure, and quality, and how to connect data initiatives to business outcomes. Scott shares insights on data governance, aligning data work with strategic goals, and the evolving roles in data leadership. This conversation is full of practical tips for executives aiming to get their organizations AI-ready through robust data management practices.
Introduction
Nate Rackiewicz: Well, hello again, my friends, I'm Nate Rackiewicz and this is your podcast of the week, FuseBytes. It's a podcast that this year is focused on AI readiness in companies. You hear about AI everywhere you go. It's at every conference that you're at. It's in the news everywhere. It's been an explosion of interest since ChatGPT burst onto the scenes about a year and a half ago. And here we are talking about AI within companies and how difficult is it to implement.
The truth is, it's not simple, even though you hear about it everywhere. And we wanted to go through in this series of podcasts called FuseBytes some of the steps that are required to get AI ready within your company and among those is getting data ready because you can't have AI without high-quality data. It's the fuel that really drives the AI algorithms. And if you don't have high-quality data, you've got ‘garbage in garbage out’ – the old saying.
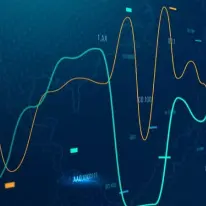
Discover how your business can grow and transform with AI.
Book a meetingSo how do we set up AI models and algorithms for success when thinking about data? And how is data for AI different than data we've worked with for the past 10, 15, 20 years, since the big data boom that happened and was being used for data analytics purposes on the predictive analytics and then into prescriptive analytics? Here we are now sitting around prescriptive analytics with AI. And how is that data different? Well, I'm excited to bring a guest to the table here today. A gentleman named Scott Taylor is joining me today! Hi, Scott!
Scott Taylor: Nate, how are you doing? Great to see you. Thanks for having me.
Nate Rackiewicz: It's great to see you again. Thanks for being here. I'm thrilled to have Scott on the show. Scott Taylor is known as the data whisperer. He's helped countless companies by enlightening business executives to the strategic value of proper data management.
As an avid business evangelist, he focuses on business alignment and the strategic why? Rather than the system implementation and the technical, how?
He shares his passion through all forms of thought leadership content, including public speaking, blogs, videos, podcasts, white papers, and believe it or not even cartoons and puppet shows. One of which I watched last night with their chief dog officer.
Accolades and recognition include DataIQ 100, he's listed by CDO Magazine as a leading data consultant, Onalytica ‘Who's Who in Data Management’, Dataversity ‘top 10 blogger’ and Thinkers360 ‘top 10 thought Leader’. He's written the book that I encourage you all to get called ‘Telling Your Data Story: Data Storytelling for Data Management’.
It's available now, Scott lives in Bridgeport, Connecticut, where he often kayaks in Black Rock Harbor, and for those that want to know he can also juggle pins and blow a square bubble.
So welcome again here to the show. Scott, how do you blow a square bubble?
Scott Taylor: I didn't blow a square bubble. That's what start from the bottom here. It's actually a bubble cube and you blow 6 bubbles that are attached to each other, and then you put a straw in the middle and when you blow there the magic happens, and a little cube appears.
Nate Rackiewicz: Imagine that.
Scott Taylor: Exactly. Yes, I saw somebody do it once on TV, and I went ‘I could do that’ when I was a kid. It was like, I can figure that out.
Nate Rackiewicz: Have you figured out the Rubik's Cube?
Scott Taylor: No, my son is a great Rubik's cuber, but I'm just a bubble cuber.
Nate Rackiewicz: Yeah, I was wondering about that as well. Well, listen we're really excited to have you on the show for this program where we're focused on the importance of data in the equation of AI readiness for companies. And nobody knows data better than you and the work that you've done in helping to educate senior management in particular about the ‘Why’ of data and why it's important and the importance of applications of that data as opposed to just getting stuck in the technical jargon that many people get stuck in.
And I think your data puppets example that you've got on Youtube that has 22,000 views is a good example of a bunch of puppets that are getting stuck in the jargon and missing the why of it all. So how did you become the data whisperer and get into this focus on consulting to senior management about the ‘Why’ of data.
Scott Taylor: I've always been a storyteller. Data storytelling now is a super hot thing, but I've been in storytelling since it was 2 words and I go back in data Pre 2K, so I've got decades of exposure to what I saw as common challenges, similar issues, common goals at enterprises at the level that you and I deal with. They're certainly more the same than they are different when it comes to some of the issues that they face with enterprise data and enterprise data management.
The data whisperer moniker was just something that hit me one day, kind of like the dog whisperer the horse whisperer, speaking data management. We calm data down, we train data, make sure data is acceptable and can be used. So it was a little bit tongue-in-cheek. I put it on a badge once at a conference, and I got so much positive feedback I never took it off again. But spoiler alert, even though I'm the data whisperer, I don't do a lot of whispering. I am out there yelling, telling, and selling about the power and value of proper data management.
You can't yell and tell and sell that any louder or stronger these days given the deluge of AI and GenAI and the hype cycle we're in which is unlike anything I've ever seen, and I've seen a lot of it.
“Spoiler alert! Even though I'm the data whisperer, I don't do a lot of whispering. I am out there yelling, telling, and selling about the power and value of proper data management.”
Nate Rackiewicz: It really is a hype cycle like none other. I thought the big data hype cycle was extreme back when we went through that in the mid-2010’s and this really just blows that out of proportion. It has everybody talking about it everywhere. You can't turn on CNBC without coming across something related to AI or Generative AI. Nvidia is all over the place as the most valuable company out there in terms of market cap. And so it's it's something that I've never experienced anything as big as is what we're going through right now as well.
Nate Rackiewicz: I'm wondering, how do you think data is changing with the world of AI. We've got data, we've been using data. We've been using big data for years for analytics purposes, organizations navigate their analytics journey of descriptive analytics, to diagnostic analytics, to predictive analytics, to prescriptive analytics. And they've been using data to do that for years. In comes AI which allows for a lot of fast tracking of things.
How does that disrupt the world of data management? And how should we be thinking about refining our approach to data management in a world of AI.
Scott Taylor: It just reinforces the need for proper data management, formal data governance, data structure, data foundation, whatever you wanna call it. I like to call where data starts versus where it ends up, which are things like AI and analytics and business intelligence and whatever the flavor of the week is.
But you can boil my entire data philosophy down to 3 words. And as a storyteller it's important to be succinct and try and get things down to a crisp level, especially for business executives that don't have time to hear about data. But my 3 word data philosophy is ‘truth before meaning’. You have to determine the truth in your data before you derive meaning. It is not chicken or egg here, it is egg and omelet. If you don't have the truth in your data you're not going to get the meaning out of it that you expect, and that hasn't changed.
“My 3-word data philosophy is ‘truth before meaning’. You have to determine the truth in your data before you derive meaning.”
I haven't found a way that that's changed. It's more important now. The scale of AI and generative AI are, staggering compared to what we looked at when we were just dealing with things like implementing enterprise systems and spreadsheets and basic business intelligence. But the fundamentals are the same. And that's part of what I try to point out, even though the glossier and frothier and sexier and hotter and more spectacular, and kids know about it, and everybody's using the fundamental aspects of it are the same.
You mentioned GIGO in your intro there. We learned that on the 1st day of data right, garbage in garbage out, sure as gravity. What goes up what must come down, and what goes in must come out.
Nate Rackiewicz: Yep.
Scott Taylor: But it reinforces what I like to call the golden rule of data, which is, do unto your data as you would have it do unto you, what you're gonna put into is what you're gonna get out of it. And for some reason, 30 years in this space, I still have to pound that message as strongly as ever.
“It reinforces what I like to call the golden rule of data, which is, do unto your data as you would have it do unto you. What you're gonna put into is what you're gonna get out of it.”
Nate Rackiewicz: So it seems that you're a big proponent of really focusing on the ‘why’ as opposed to the ‘how’ and it seems that the ‘why’ is changing. Because the needs of that data are changing. So why we have that data and what we're doing with it is changing in the world of AI from where we were before and helping accelerate, you know, predictive and prescriptive analytics as well as everything else that it's doing.
Nate Rackiewicz: How do you advise, senior management in companies that you go into or talk with at conferences? About how that ‘Why’ is changing around that and what is the reception that you tend to get from those executives that you speak with?
Scott Taylor: I don't know if the ‘why’ is really changing as much as maybe it appears the stakes are different. The speed is different. The scale is different. The expense is different, the players are a lot more, the effects can be much more widespread, but the essence of the ‘why’ is based on my belief that every company is trying to provide value to their relationships through their brands at scale.
That's what they want to do. Generative AI makes that scale a lot more exciting and a lot more expandable, but it's still the same thing. Again at its essence boil it down, so the why that I reinforce is why does what you want to do with data fill in the blanks as to what the current hype cycle is telling you. Why does that enable the strategic intentions of your enterprise? Where is your company going? And why does data help you get there?
And taking that apart at an organization I look at 2 basic buckets. If you go back to that statement providing value to your relationships with your brands at scale, relationships every company has relationships. You don't have relationships. You don't have a business.
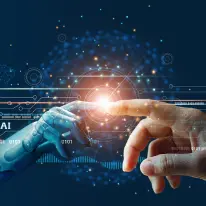
Partner with Fusemachines & unlock the transformative power of AI.
CONTACT US TODAYNate Rackiewicz: Right.
Scott Taylor: The data about those relationships is as critical as any data you've got. And then every company has brands, products, services, offerings, whatever they might be. But that's how they provide that value to those relationships, and that keeps me really steady in an exercise I do, which is anything new that comes up, I find, where that is. Where's the truth before meaning hook? Where's the foundational need? Where's the reinforcement of providing value to relationships with their brands at scale. So I'm not a trends guy, so I don't look up at what's new, but I wait till what's new shows up, and then I go– Okay, how do I fit this into what I see are inextricable, undeniable laws of data in an enterprise.
Nate Rackiewicz: So that's really interesting. And I love when you talk about storytelling as well. And your book really focuses on data storytelling and data management. I wonder over the past year and a half what are some of the new stories that have emerged that you find yourself talking about at these conferences, in your blogs, in your consulting opportunities with these executives. What are some of those key stories that are emerging?
I saw the data puppets was one that was focusing on the chief dog officer instead of the chief data officer arguing with the chief information officer and all of them, forgetting the fact that the monthly reports weren't even working so they were caught up in all the jargon and things like that. How would you? And and I think that that was created about 2 years ago. If I if memory serves me correctly.
Scott Taylor: Yeah, yeah.
Nate Rackiewicz: If you were to create data puppets for today and with an AI slant with all of the stories that you've seen and are emerging. You know. What might that look like today?
Scott Taylor: It again. It doesn't look so different. I do have another, an updated data pup that I'm working on an epic multi part series with the data puppets. If anybody's interested in finding them just simply Google, the term data put that's it.
Nate Rackiewicz: They're great because they simplify. You know, some of the main things into a story that people can understand.
Scott Taylor: The most common feedback I get is that's just like my company. I was just in that conversation, and you're talking about a monkey and a bee and a dog and a cat, and they're arguing, and they are literal hand puppets and it seems to strike a chord with folks because the chief CDO, the chief dog officer, he's struggling to convince and manage data in his organization. He's just being bombarded with every type of of problem that people show up within the latest one.
It's a preview of this multi-part series called Journey to the center of the single version of the truth, and he meets up with a cat Sultan from Meow Kinsey, who only wants to buy more software. He meets up with a couple of software vendors, sales, fork, and micro spoon, and so there's culinary utensil theme in there. There's a puppy intern that only wants snacks, so he doesn't care about what work he's doing. Then he runs off to go at Arf B&B. So it's definitely full of infused with dad jokes in the data space, but definitely resonates with folks. And you just mentioned, this is my book. So everybody knows what it is just mentioned telling your data story data storytelling for data management, 99% buzzword free.
I didn't want to overpromise. And it takes people through this, I still think a very common journey of how to explain why telling a story about your data's importance is as critical as ever.
I joked with my publisher to just ‘update’. It was written during Covid so it came out the end of 2020. It's like, okay, we could update this. I'll just throw GenAI in there every 3rd paragraph, and it's still the same thing. I think that would decrease the buzzword free count, however. The new stories that people are telling they're just more enhanced. They're a little more urgent. They're a little more concerned.
They see this double edged sword of with GenAI Data can literally run away from you in ways that it hasn't before, where you couldn't contain it, and a lot of folks are still struggling with that.
“GenAI came out and everybody was, Oh, wow! This is fantastic, and all of a sudden not too much longer after that they started talking about the need for a proper training corpus. We need AI governance which just doesn't cover the models, but it covers the inputs.”
This is a common story. You go from GenAI all the way back to General Ledger. When people were said we need a chart of accounts, and you're gonna find these common themes in there.
Nate Rackiewicz: One of the things that we think about with big data and LLMs is concern about bias in the data, concern about the sensitivity of data that you're using to train those models also getting out. How do you think about that? In the concept of the storytelling that you do with senior management about the ‘why’ of the data as it relates to concerns that they hear about the board hears about. Oh, I should be concerned about bias in my data. So I don't want to adopt AI.
Scott Taylor: If you find the truth in that data, and when I say truth, I don't mean anything philosophical or political, it can really structure data around let's say, your customer file, your customer hierarchies, your product categories, your product distribution, the fundamental piece parts of your business, when you have that really squared away, and in a shape that people are confident that they can believe in it.That should do a lot to influence the lack of bias in whatever result, you're getting.
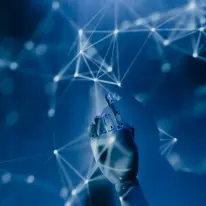
Leverage AI to elevate your operations and gain a competitive advantage for your business.
Book a meetingThe security part. I'm not a security expert, so you need system folks to put that in there, but getting too far off of the truth of what you're trying to find meaning in starts to happen when you have things as simple as conflicting hierarchies or lots of duplicates in your relationship data or hierarchies that don't make sense or geographies where people have different definitions.
An exercise I used to take people through is ‘How do you define Chicago?’ and you've got media markets and scanner markets and sales markets and measurement markets, lots of different configurations of something that's called the same thing. And that along my theme of problems that have always been there, but that's exacerbated. If you start to put these LLMs on top of it, you may pick the wrong one without knowing. So I think a lot of it still comes back to some form of data governance, some form of data management, some form of stewardship, some form of agreement around the organization about standards.
Everybody knows what a standard is. Standards work all across organizations and industries and markets. And so that concept for me helps explain why it's important to invest in this.
“And the ‘why’ that I focus on is, why should the business care? Why should the business care about the work you're trying to do in data management? Why should they care so much that they can give you money that they could spend on things that frankly appear and end up being more tangible usually than data management, data stewardship and so on.”
So it gets back to that, starting off the right way.
Nate Rackiewicz: So you've mentioned both data governance and AI governance. Do you see the 2 of them working together? Or do you think that they're distinct entities within a company in terms of how they should be handled? Should they be one in the same in your opinion or do you think that they do need to operate independently.
Scott Taylor: I think that they have to be as close together as possible. I think the scope of what AI governance is still in discussion and being defined. But AI governance certainly includes the inputs as well as the models, and probably the distribution and a few other things. But they've got to be as close together as possible. I always struggle when data science was really hot and people kept talking about how data scientists spend 80% of their time munging and wrangling data. And then the joke was, they spend the other 20% complaining about munging and wrangling data.
But it struck me and I asked around and it seemed to be true. Why isn't that team talking to the data governance team, who knows the data management team, who knows where a lot of that data is, who knows which data is good and which isn't? Who can probably help you avoid some munging and wrangling.
So there's this bifurcation in the data space, if you will, between the folks who manage the data and the folks who use the data, between data management and business intelligence analytics. There's always been this separation that for me is extremely frustrating. And I hope that AI governance doesn't get too far away and becomes its own department versus data governance. Because these things are so inextricably linked.
Nate Rackiewicz: Yeah. And I rode that curve there with the data scientists through the 80% problem of data wrangling and the 20% of complaining. And I see the same thing emerging to a degree with AI. Data science has really evolved to a degree into AI and it's the data scientists that were kind of at the leading edge of tackling the new AI algorithms and have become the AI engineers in many cases that we see today.
But the AI engineers are often left to themselves, separate from the data engineers, let's say. And so that's a common pattern that I see in companies that those two are really distinct. And the AI engineers end up doing their own data annotation, they do end up doing their own data, wrangling, their own ETL work in some cases, because they're not connecting those dots that you talked about. And I think that for a company to be AI ready, it really is important that we fuse those things together so that they can be more seamless and work together. But what does that mean in terms of governance? I think it is up in the air, in my opinion, around AI governance like you said so I agree with you on that, too.
Scott Taylor: I know you're into fusing. So that's good. How's that?
Nate Rackiewicz: Yes, fusing as a team, fusing with machines over here at FuseBytes.
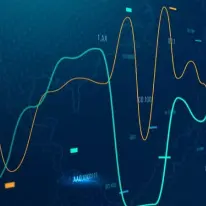
Discover how your business can grow and transform with AI.
Book a meetingScott Taylor: Yes.
Nate Rackiewicz: So you speak about some of the fundamentals that people know. What are some of the fundamentals of data governance that you think need to be in place for any company that is looking to tackle the data problem and the ‘why’ of data governance head on and recognizing that it is supportive of both traditional analytics as well as AI? What would you advise companies to put in place with data governance so that they don't end up with the chief dog officer problem, where they're just talking about buzzwords and not actually getting anything done?
Scott Taylor: Not speaking as an organizational expert, but just as somebody who has lived in the space for as long as I have, data governance needs to be able to tell the story of why it's an important part of the organization and provides horizontal value across everything an organization does. And when I define data governance. I'm gonna split the hairs between data governance, data management, data stewardship, data quality, these are all parts of that high level the phylum level, if you will, of data management versus business intelligence, and both of those tend to roll up into just a thing called data or data and analytics and data management. It also includes pipelines, data observability, things that don't have to do with quality. But for me to simplify it, I just say, okay, 2 big buckets here, getting the data ready where data starts and using the data where data ends up.
And there's a little bit of a gray area in between. But big things happen on both sides, and so, making sure you align those efforts and get the stakeholder engagement, get the C-level attention, getting in the room somewhere when a lot of these big ideas are happening that are technology based, that's the clue. If they're gonna use technology in your organization, there's gonna be hardware, there's gonna be software, and there's gonna be data and if there's data, there's gonna be data management.
So trying to make sure you get into those rooms where it happens is critical. And it's still a challenge because data governance data management that whole bucket tends to be relatively boring. It's never really sexy. It's clerical. It's back office, it's you know, things like MDM and reference data management sound like that. They've been around for a long time. Are those really thrilling? You know, we want to do GenAI.
“But when people say that it's like– Oh, I want to do GenAI, but I don't care about data management. That's like saying, I want to have a great meal, but I don't care about the ingredients.”
Now you can get somebody to cook the ingredients, cook the meal for you but you hope that they care about the ingredients. Food is one of my favorite analogy sets to use when you get into the data management space because everybody's got to eat. And it's relatable to everyone.
Nate Rackiewicz: And I'm looking forward to the fork and the spoon analogies coming out with your next puppets.
Scott Taylor: Yeah. So for me it's really just important to keep pounding that message and the reason I wrote my book and the reason I do a lot of the work I do is because I had met so many data leaders focused on the data management side, who had this dual emotional state where they were really passionate and devoted around what they believed data could do for their organization, but they're really frustrated because people didn't listen to them.
Nate Rackiewicz: Yeah. Well, and why do you think that is that people didn't listen to them? How were they not successful in getting through?
Scott Taylor: They tell a terrible story they explain it with, and this is the same for all. I'll throw this cast across most data people, if you will. They love to start with how they did it.
Okay, first we did this, then we tried this, then that didn't work. So then we tried this, and you know we've got the latest that. And then these guys came in and showed us that this will be even better, and you've lost them already. They don't care. Tell a story, but don't tell your life story. I don't need to start with, I started off as a child, and then I grew up. You gotta get to the point. And the story you tell about data when I present data storytelling the type of story it is, is a pitch, it really is, and it's a sales pitch. I got no problem with selling. I'm 4th generation sales and proud of it.
Selling is the way to convince somebody of the benefits of what you have to offer. It makes things happen. So all the good parts of selling I think people should really focus on. But you need to be able to get somebody to say, yes, that's what you want, right? You wanted to go from “I have no idea what you're talking about” to “How do we live without this?”
Nate Rackiewicz: Yeah. I think that's really a great point. So the blend of data, if data were a big mixer bowl you know, in your food analogies, you've got your first party data, sometimes your second party data, and then oftentimes you've got third party data.
How do you think about when you start to blend those data sources together? Different ways that you have to treat those different types of data first party, second party, third party data sources.
Do you think about those in different ways? Do you think that they need to be treated in a different way as you're going through the governance process so that you can ensure higher quality for things that you may not be as in control of like third party data. Because first party data, it's entirely your responsibility. Second party data you've got a little bit more control over, third party data you're getting what you're getting and as my wife likes to say, you get what you get and you don't get upset, with these things. And so how do you think that translates into data governance relevance? And you know ultimately into that data feeding these AI algorithms so that an organization can be AI ready?
Scott Taylor: My whole career was based on representing 3rd party data sources, as they would probably be categorized now. So 15 years at Nielsen, I was at Dun & Bradstreet. I consulted for Kantar, WPP, these world-class iconic data providers who provided content to enterprises to enrich and enhance and structure the internal data they had.
This was before the parties started to get identified. But again if it's not exactly the same, it's certainly more the same than different in today's world.
So you've got that 1st party data which you know, your master data reference data, your internal highly structured, well governed, expertly stewarded source of truth in whatever configuration fashion you can make it happen.
“You gotta set that standard in your organization, and nobody's ever convinced me that you can't. They've convinced me that they can't get it done but they haven't convinced me that they shouldn't do it.”
Nate Rackiewicz: Right.
Scott Taylor: When I was at 3rd party suppliers. A lot of what the parts of the business I was in actually were, the ones that help people align and integrate and standardize their data with our external data. So Nielsen had a set of store records, grocery store records, we found a way to use a unique identifier on these records to map it to the internal customer masters of these consumer packaging companies.
So everybody who sells something that goes into somebody's kitchen or bathroom or house at any of the you know, grocery stores, supermarkets, mass version sizes, and so on. You know, all those suppliers have a different version of that store record and they're trying to integrate disparate data sources around either a store or an account or a market or some one of these different dimensions and so that alignment I worked on helping people syndicate their deliverables so they could integrate more seamlessly with internal data of the client side, on the enterprise side.
It was fascinating. I love that. And that's where I started, and I've always loved that part of the space, because again, those challenges, the scope of them are much bigger. The scale is huge, the speeds incredible, but the challenge is still inherently the same. You got lots of columns of data. You gotta align them to the rows. Columns are easy to add. Rows are hard, so anybody who's tried to, put spreadsheets together—
Nate Rackiewicz: Yeah, the problem comes in the rows with bad data.
Scott Taylor: Yeah, if the rows are bad, then the columns don't mean anything. It's like the rows are master data, the columns are analytics.
Nate Rackiewicz: You talk about trust, right? How do you evaluate the trustworthiness of 3rd party data as you're allowing it to enter your system?
I've been, more on the recipient side of 3rd party data sources in my career rather than on the supplier side. And so, I've seen those bad rows coming in. And I wonder how you think about evaluating the trustworthiness of that data from the perspective of a company that is ingesting it and merging it in the grand mixing bowl that they've got in their kitchen.
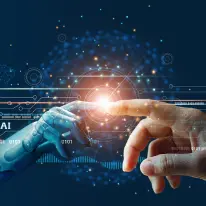
Partner with Fusemachines & unlock the transformative power of AI.
CONTACT US TODAYScott Taylor: Everything. There's more 3rd party data sources now than ever before. There's probably more now than there were when we started this podcast. And everybody thinks their stuff is, you know, absolutely fantastic. This is it, high quality, this is gonna give you the insight you need. When you're an enterprise thinking about it. I think you want to think about a couple of areas: coverage, structure, can it connect? Even before you think of quality, Does this cover my business? Is this whatever this source is, is it about something that I care about? And is there enough of it?
Does the scope of whatever this syndicated data provider has, does it cover enough of my business? If you get the most incredible, fantastic data about 3 census tracks or a couple of block groups, or a really sort of unique situation.
That's great for a proof of concept. But I can't put that in production. I can't run my whole business off that. I can't have my national sales force getting insight that's gonna help them develop customer relationships if I only have like this little piece of it. So, understanding that coverage. I worked at Dun & Bradstreet? They have, I don't know half a billion records.
Nobody needs all of it. So it was that kind of discussion. Then you wanna get into some form of structure. Understand the structure of that data that you're getting. Does it have a unique identifier that you could map to yours? As simple as that. That hasn't gone away. People haven't figured out ‘Oh, we don't need unique identifiers.’ But those tend to have to connect somehow. At what level? What level is a hierarchy. Going to things like products and retailers are simple. Organizations are easier to describe than consumer data or people data.
Nate Rackiewicz: Yeah.
Scott Taylor: But organizational data, they may have a branch, they may have regional headquarters. They may have a parent office in the country, they may have a global ultimate parent. What level do you even work at? And on the enterprise side, which of those levels are important to you?
It could be on a product a brand information, it could be a skew, it could be all the way up to, a brand franchise or a global brand, if you look at Coca-Cola, it's a global brand name, but then it splits up into flavors and it goes way down to the SKU level of Is it a bottle? Is it a can? Is it a 6 ounce? Is it 12 ounce? Those all are either really meaningful or completely worthless depending on the situation.
So coverage, connectability structure and then you get into things like quality. And for me, quality is when I counsel people, even on the enterprise side to sell a data governance program. I beg them not to lead with quality. Quality is a really emotional subjective term. Everybody's got a different definition of quality.
You could give data to somebody, and they find 2 mistakes in a million records, and those 2 mistakes are things that are really close to them for some reason, and they think the whole file is garbage there's lots of bigger, deeper thinkers into how to assess quality and it's important.
But for me getting back to what I do every day, which is help people sell this stuff in. Don't lead with that. And the proof that it doesn't work, to lead with that is, we've been talking about it for decades and we still have challenges convincing people it's important to invest in. Nobody’s gonna say, bad quality is a good idea. But also no CEO in their annual report letter to their shareholders, is going to write about how next year's significant initiative includes better data quality.
Nate Rackiewicz: Yeah.
Scott Taylor: So how do you map those 2 things, you gotta connect that.
Nate Rackiewicz: Yeah. And how do you connect it to the ROI? Right?
Scott Taylor: So I connect it that way and go, Okay, read the rest of that CEO letter. Watch the rest of that presentation from your senior leadership that they did on investor day. Watch their interview on CNBC. Listen to your analyst calls. If you're a public company, find out what they're questioning. Hear that. What's your strategy day? What's your company vision?
You're gonna find the hints in there. You're gonna hear about relationships. We want to be the premier partner of choice for our customers. We want to expand to different sectors. We need to find deeper, valuable ways to bring value to our relationships, to our brands at scale, different versions of it. It's gonna be in there.
And the way they're gonna do it, they're gonna talk about their products and their brands and their services. So listen to those things and all that high level strategic stuff. It's your job at the organization to connect it with the data work you have to do.
Nate Rackiewicz: Yup, and to demonstrate that through business outcome based solutions. One of the things that I like to say is that you've got to focus on the corporate North Star. What is it that we even care about data for? What are we trying to accomplish?
And then what are the business questions that you have to ask on the ladder? And it's a ladder of business questions that you have to ask to get to that corporate north star. You know, questions beget questions. And so these ladders form that you're trying to achieve. And those business questions themselves can become use cases that can then be implemented with data, governance, with analytics, with artificial intelligence as well in order to satisfy answers to those business questions.
And so I like to lead with that business question based outcome that you're trying to achieve so that it's clearly tied to something tangible that the organization cares about and isn't just doing technology for technology's sake, and because it's the next big thing from a technology perspective.
Scott Taylor: Another very tactical but very impactful approach is making sure you've got examples of really horrible data that the work you are proposing is going to help fix because people don't always see the stuff behind the scenes. Really early in my career I was in the sales organization, presenting to them. The head of sales just goes, Scott we can slice and dice our data any way we want to, all I have to do is push a button and this really courageous data analyst at the end of the table just raises like ‘Sir. I'm the button’. People have to deal with that pain.
Nate Rackiewicz: The data munging that goes on.
Scott Taylor: Data munching, data wrangling whatever it is. And I have a slide of my presentation that I use always and it's an example of 275 ways an organization spelled 7-11. And people just they love it. I tried for a while to not use it, but it's like one of my greatest hits and the exciting thing is it’s still relevant, people still get it. But the depressing thing is, it's still relevant, people still need to see that cause it's 30 years later. But find an example like that I mean, there's a point where you gotta rub their nose in it in the right way in the right context.
But you've gotta show them where the pain is. You have all these wonderful aspirations that are going to happen based on data. We get that we know we can help you or based on GenAI or whatever you want. But look at what we're dealing with back to how do you trust it?
Look at this mess. Does our sales force even understand the concept of searching before they create another record? Is there any way we can help set standards? We got 22 places to create customer in our organization. These are not uncommon problems, and the bigger the enterprise, the worse it is, and the more an enterprise has grown by mergers and acquisitions.
Nate Rackiewicz: Oh, yeah.
Scott Taylor: The more it's like. You know, people start to give up in those situations.
Nate Rackiewicz: I've seen that first hand. And we see it with customers, clients as as they're going through these data needs that they have to have solved. It's oftentimes the comments are, the data lake and data lake house are neither a data lake nor a data lake house nor data warehouse. It's just a bunch of crap and crap is the nice word. I was on a call earlier today talking about data and the concept of what we have at this company is a dumpster fire.
And so this realm of getting that in front of people that are decision makers to understand the implication of having a dumpster fire of data so that you can make the business case for that, because that dumpster fire is what you've got to train your AI models and you wanna become an AI ready company. And so how do you make sure that you can do that?
I think it comes back to the business case, the business question that's being asked, mapping that to the AI problem that you're trying to solve from an AI perspective saying, Okay, what is the data that I need in order to solve this problem and then tracking that back up and going through standards of understanding what layers of governance are there around that data and working it in that reverse engineering way and trying to take a look at the data that you need and understand the full data lifecycle management, that data governance is responsible for that before really jumping fully into creating the AI algorithms. Assuming that the data is good enough to work with.
“You've got to really follow that pattern of tying it to business outcomes, focusing on the algorithms, asking yourself, what data do I need? Taking a look at the data, making sure that it's governed and understanding that which gets back to what you were saying before about bringing together the AI engineering with the data governance much more closely, so that, that conversation can happen.”
Scott Taylor: It's gonna happen somewhere. It's either gonna happen and get solved or there's something's gonna break later that's gonna be a big mess that they're gonna have to piece apart and realize. Yeah, I mean, most of the time, it's the data's problem. The data's fault.
Right? Software works, software works, hardware! do they have big hardware problems anymore? Right? Everybody's got cloud to put plenty of storage. So those kinds of other than little, minor glitches is not what I'm talking about. But usually the data was the problem. It gave them the wrong answer. It skewed it the wrong way. There was bias in the data. There was some kind of missing piece that people didn't see, or multiple versions of the same thing. That tends to be more of the more the issue.
So, fixing all that stuff earlier, getting your handle on it, just getting into the conversation that this is part of it, I mean, that's all I'm really ever asking for. It's like, just get, as I said, get us in the room. Make us part of it. I remember this when ERP and CRM were starting to roll out, was big thing, and they would, Siebel and SAP and so these early brands that were in there, and it just brought to light all these enterprises that would just, buy new software everything, Demos beautifully cause it's not your data in the demo and then they try to start that car up and the gases got sand in it to push the analogy and dealing with data people who were just like, I wish they'd let us know when they put us in there.
Nate Rackiewicz: Yeah.
Scott Taylor: For the satisfaction of some of this, I think, has come, which is a really good development, at least in the idea of a chief data officer of somebody who is accountable for the data. Not necessarily the technology, I'm mildly disappointed is the CDO titles have expanded the CDAO. CDAI- OU, sometimes Y, or whatever way they just. You know, we get in our own way so much.
Nate Rackiewicz: Sure.
Scott Taylor: In terms of some of this. I mean I don't know about you, but I don't know how many c-level spots there are that you could divide up a CDA, a CDO, a CAO, and a CAIO. I don't think there's a lot of companies who have that kind of room around the board table.
Nate Rackiewicz: Right? Yeah. I mean, I served as a chief data officer prior to joining Fusemachines. And it was, you know, for a company that went through a big set of mergers and had, an integration challenge that needed to be faced and there was, a strong need for an urgency around that, and I think that the titles derive from the urgency that's created within that company and what is core to that company at a given point in time,to really kind of say, this is the emphasis that we wanna put on getting this right.
And in some cases it might be a chief AI officer. It might be an AI driven company that really needs that, because that's quarter their brand and it's part of their core competency that they're looking to expand. But you know, I think here's often confusion about where should AI sit within a company?
Should it sit under the chief data officer? Should it sit under the chief technology officer, should it sit under a chief AI officer? And you see it in different places at different companies and I think that where it sits is based on something that's growing organically over time, mostly. But ends up being in the place that it can have the most impact within the business.
Scott Taylor: I just look at it structurally and go. Okay, is there room for? I guess I'm mildly cynical on some of this surprise surprise. But it's like it, you know. It's there's a CFO, but there's not a CARO and a CAPO. Right. It's just like there's a chief marketing officer, but there isn't a chief TV officer and a chief website officer. I mean, just at some point you gotta stop. And I just wonder how that sounds to the rest of the organization.
We live in a data bubble and an exercise I encourage people to do is is pretend you're a different part of the organization, and say some of this stuff, and see if it even makes sense at all. We spent so much time in the data space pontificating around the existential concepts of how do we bring value? And I don't think other departments do that much. I don't think the Finance departments wondering how it brings value to the business every day.
Nate Rackiewicz: Right.
Scott Taylor: Or the marketing department, they get it done. They have struggles, they have hype they got buzzwords, they got craziness, too, but there's just something around the data, IT, analytics space. Again, I feel like we get in our own way a lot of way times. And I think the way we talk about data is holding the industry back, which is one of my core concepts that I preach about all the time.
“Just talk about a business-accessible way. Get the work done. Come up with the business reason, not the data reason. And part of that is communicating in a way that people understand you.”
Nate Rackiewicz: Yeah, it's the magic of the storytelling that has to happen. That storytelling has to happen around the why as opposed to the how?
Nate Rackiewicz: Well, listen, Scott. We're approaching time here. And I wanna thank you for joining us on FuseBytes. I thought this was a really productive conversation. I thought your insights were really valuable. I heard really great sound bites as we went through with really good tips and tricks that are in there. And I hope that our executives that are listening to this program will take those, write those down and implement them in their own firms as they think about getting AI ready and making sure that they're data ready so that they can be AI ready. But it's been really a pleasure having you today here, Scott.
Scott Taylor: It's been great. Nate. Thanks for having me. This was fun. Time went right by, and again people follow me on Linkedin. I've got a Youtube channel where all my videos are, my book telling your data stories on Amazon and reach out to me on Linkedin if you ever wanna connect on anything. So thanks again, though, thanks for having me.
Nate Rackiewicz: Sounds great and thank you all to those that are listening to this podcast so we really appreciate you. This is FuseBytes and I'm your host, Nate Rackiewicz, and we'll see you on the on the next episode.
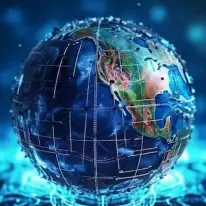
Leverage our AI products and solutions.
Schedule a meetingGet to know our speakers
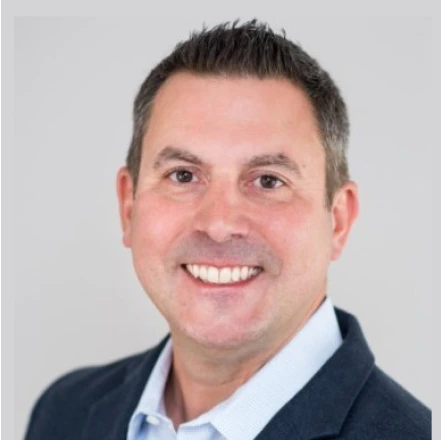